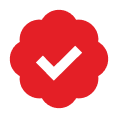
Estimated reading time: 9 minutes
most relevant
Trying to wrap your head around the difference between data science and computer science?
Many of the luxuries that we have today — a favorite streaming service that recommends new movies, the ability to unlock our phones with facial recognition technology, or virtual home assistants that let us play our favorite music just by speaking — are made possible by computer science and made better by data science.
Today, these two fields complement each other to further applications of artificial intelligence, machine learning, and business forecasting. Read on to learn a definition of each, their histories and applications, and career paths in computer science and data science.
What Is Computer Science, And Why is Computer Science Important?
Computer science is a broad field of study involving both theoretical and practical topics like data structures and algorithms, hardware and software, and information processing. It’s an umbrella field with many subdomains and applications, such as machine learning, software engineering, and systems engineering.
Computing devices such as the abacus date back thousands of years, but it might surprise you to discover that the field of modern computer science is nearly 200 years old. Ada Lovelace pioneered the field of computer science by writing the first computer algorithm in the 1840s.
Computer science is important because it lays the foundation for how technology and digital systems are created. Technology permeates every aspect of our personal and business lives. Each household has an average of 22 connected devices, according to Deloitte. Transformative technology, such as life-saving diagnostic tools, online learning, and remote work tools, are all built with computer science.
What Is Data Science?
Data science is an interdisciplinary field that uses data to extract insights and inform decisions. It’s often referred to as a combination of statistics, business acumen, and computer science. Data scientists clean, explore, analyze, and model with data using programming languages such as Python and R, along with techniques such as statistical models, machine learning, and deep learning.
While many of the topics used in data science have been around for a while, data science is still in its infancy. In 1974, Peter Naur defined the term “data science” in his work, Concise Survey of Computer Methods. However, even Naur couldn’t have predicted the vast amount of data that our modern world would generate daily only a few decades later.
It wasn’t until the early 2000s that data science was recognized as its own field. It gained popularity in the early 2010s, leading to the field as we know it today — a blend of statistics and computer science to drive insights and make data-driven business decisions. “Data science,” “big data,” “artificial intelligence,” “machine learning,” and “deep learning” have all become major business drivers today.
One reason that data science has grown in popularity is that historically, we did not have the storage capacity to collect and store the amount of data we can today. While many of the tools that data scientists use have been around for many years, we did not have the software or hardware requirements to use these tools until recently. The emergence of big data and technological advancements has paved the way for individuals and businesses to harness the power of data.
Data Science vs. Computer Science
The study and use of data – information such as numbers, text, and images – has applications in both computer science and data science. Data structures are ways to organize, manage, and store data to use it efficiently. As a sub-domain of computer science, data science allows us to access data in a computer’s memory. Data science benefits from data structures to access data, but the main goal of data science is to analyze and make decisions based on the data, often using statistics and machine learning.
The career paths of each of these fields differ as well. Computer science is used in many different jobs, including data scientist. Other common roles involving computer science skills include software engineer, computer engineer, software developer, and web developer.
Since computer science is one component of data science, there is often a crossover in these roles and responsibilities. For example, computer science tasks like programming and debugging are used in computer science and data science jobs. Both of these fields are highly technical and require knowledge of data structures and algorithms. However, the depth of this knowledge required for computer science vs. data science varies.
Think of it this way: data scientists know more about statistics than computer scientists but more about computer science than statisticians. These interdisciplinary fields interact with each other in the workplace. It is also noted that while computer science is “deterministic”, data science is “probabilitistic.” Decisions in data science are based more on likelihood.
The Future Of Data Science And Computer Science
Today, all companies and industries can benefit from both fields. Computer scientists drive business value by developing software and tools, while data scientists drive business value by answering questions and making decisions based on data.
Here are a few exciting advancements that will impact computer science and data science in the coming years.
- Artificial intelligence (AI) and machine learning are algorithms trained on vast data sets that can be paired with chatbots and other interfaces to allow humans to ask questions and create solutions. While data scientists are indispensable for developing AI and machine learning, they will also reap the rewards of AI to help them better perform their jobs, interpret data, and forecast results for companies.
- Quantum computing is the ability of powerful computers that use quantum bits to make calculations concurrently and much more quickly than traditional computers. Quantum computing will allow data scientists to process simulations and big data analysis in a shorter timeframe and will have applications in cryptography, environmental forecasting, and more.
- Blockchain technology is a technology for storing secure and decentralized digital records, which has applications in cryptocurrencies, banking, and healthcare. As digitized devices and products grow in popularity, cybersecurity concerns follow. Blockchain technology opens the door for technological advances to keep healthcare records, banking, and proprietary information more secure.
- Virtual reality (VR) and augmented reality (AR) are technologies that enable interactions in an immersed virtual environment or a blended virtual and real-world environment. While we often think of VR and AR in the context of entertainment or learning, there are new possibilities for data scientists to create interactive data visualizations in VR or AR environments.
As software continues to amass data and integrate with our lives and daily routines, the demand for computer science and data science skills will continue to grow. Both fields are constantly evolving as technology advances, and both computer scientists and data scientists need to stay current with the latest tools, methods, and technologies.
Education And Career Opportunities In Computer And Data Science
If you’re interested in joining these fields, the good news is that you don’t need a computer science degree.
A study by ESMI found that 31% of high-skill occupations experienced material degree resets in the past few years. Essentially, that means that employers like IBM and Accenture are lowering or removing degree requirements across many tech positions. In 2023, Hired found that 64% of employers actively recruit engineering candidates without degrees. Because of this reset, an additional 1.4 million jobs will open to workers without degrees in the next five years.
Bootcamps, certificate programs, and reskilling programs offered by employers make these fields accessible to newcomers.
Computer Science Careers
While computer science jobs are popular, there are very few roles titled “computer scientist”. Instead, computer science jobs typically specialize in areas like front-end, back-end, or full-stack.
A whopping 8 of the top 10 roles in Glassdoor’s 50 Best Jobs in America 2022 use computer science as a critical component of their job. Let’s look at these roles ranked for their high pay, job satisfaction, and number of job openings.
- Enterprise architect
- Full-stack engineer
- Data scientist
- DevOps engineer
- Machine learning engineer
- Data engineer
- Software engineer
- Java developer
Common computer science functions performed in a job include writing, testing, and debugging code, developing software, and designing applications. Individuals who use computer science in their roles often create new software and web applications.
While many of these roles no longer require degrees, candidates do need to bring high-caliber technical and soft skills into a role. They need to have excellent problem-solving skills and be able to write code in programming languages such as Python, Ruby, JavaScript, Java, or C#. They must also have a fundamental understanding of how these languages work and be well-versed in object-oriented programming (OOP).
Computer science certificate programs focus on specialty areas like Software engineering, JavaScript development, React development, or Python programming.
Is Computer Science Hard To Learn?
Computer science sounds intimidating, but it isn’t hard to learn. Anyone can learn to code if they have an eye for detail and enjoy identifying patterns, solving problems, and collaborating with a team.
Data Science Careers
Data science is applied in job titles such as data scientist, data analyst, machine learning engineer, and data engineer. Data scientist and Data engineer ranked third and seventh, respectively, on Glassdoor’s 50 Best Jobs in America 2022. Individuals in these roles come from various backgrounds, including computer science, statistics, and mathematics.
Common data science functions include cleaning and exploring data, extracting insights from data, and building and optimizing machine learning (statistical) models. Data scientists analyze and reach conclusions based on data. They need to be well-versed in statistics and mathematics topics, including linear algebra and calculus as programming languages such as Python or R, and SQL. They also need to have excellent communication skills as they are often presenting insights, data visualizations, and recommendations to stakeholders.
Is Data Science Hard to Learn?
If you take an analytical approach to problems and find statistics and probability interesting, you can learn the principles of data science. You don’t need an advanced math or computer science background to succeed.
Data Science vs. Computer Science Salary
Once you have data science and computer science skills, you can command a high salary due to the skills shortages for both. Here are U.S. salaries for 15 common data science and computer science jobs, according to Glassdoor.
Job title | Median base pay (U.S.) |
Enterprise architect | $144,997 |
Machine learning engineer | $130,489 |
Front end engineer | $129,548 |
DevOps engineer | $120,095 |
Data scientist | $120,000 |
Cloud engineer | $118,999 |
Information security engineer | $116,919 |
Software engineer | $116,638 |
Data engineer | $113,960 |
Back-end engineer | $112,384 |
Java developer | $107,099 |
Full-stack engineer | $101,794 |
Systems analyst | $87,255 |
Web developer | $82,484 |
Data analyst | $70,176 |
How To Become A Data Scientist Without A Degree
With the rapid acceleration of technology, particularly in data science, there’s a high demand for professionals who can manage data sets, build data structures, and interpret and visualize data.
To become a data scientist without a degree, you need to demonstrate a foundation in statistics and probability, programming languages such as Python orR, and SQL, and communication and presentation skills. The best way to learn these very specific theoretical and technical skills quickly is with a data science certificate program or boot camp.
A data science boot camp combines online or in-person learning, hands-on projects, and expert mentoring to teach the basics of data science in a bootcamp environment. The result? Individuals complete the program as job-market-ready data scientists in as little as 12 weeks– with a capstone project and alumni network to set them apart in their job search.
Still deciding which science is your science? Hear from experts by registering for an upcoming info session.